AI-Based Support System for Chest X-ray Report Annotation - Exploring Annotator Performance Across Experience Levels
Project by Lea Marie Pehrson
The project is a preliminary step in the development of an AI-based support system for chest X-ray report annotation, leveraging input from both radiologists and non-radiologists with varying levels of experience as an alternative when radiologists are unavailable. For developing the system, a study was conducted to explore how these different annotators annotate 200 chest X-ray reports. The goal is to investigate the performance and agreement between different annotators in annotating chest X-ray text reports. The aim of the project is to investigate the performance and agreement between these annotators in annotating chest X-ray text reports.
Project BackgroundChest X-rays (CXRs) are the most performed diagnostic image modality. Based on technological advancements, there has been increased interest in improving radiologists’ efficiency and accuracy, particularly through AI-based systems for annotating findings on CXRs. These systems require training and testing using labeled data.
Ideally, CXR training data should be manually labeled, but this process is time-consuming and expensive. Therefore, systems for automatic extractions of labels from CXT text reports have been developed. These extracted labels are lined to the corresponding images, creating large labeled datasets at lower cost and time.
This project investigates how varying levels of radiological experience impact reading comprehension and annotation performance on CXR text reports. The Matthews Correlation Coefficient (MCC) was used to assess annotator performance and compare accuracy against "gold standard" labels, which were annotated by experienced radiologists. Results show that more experienced radiologists perform better, as reflected in their higher MCC scores.
Project PotentialAutomating the annotation af CXR data has significant potential. Artificial intelligence can streamline the process, reducing both time and cost by extracting labels from existing CXR text reports. The resulting annotated dataset can be used to train and validate deep learning models to assist non-radiologists in their work.
Conceptual Overview of the Chest X-ray Text Report Annotation Process
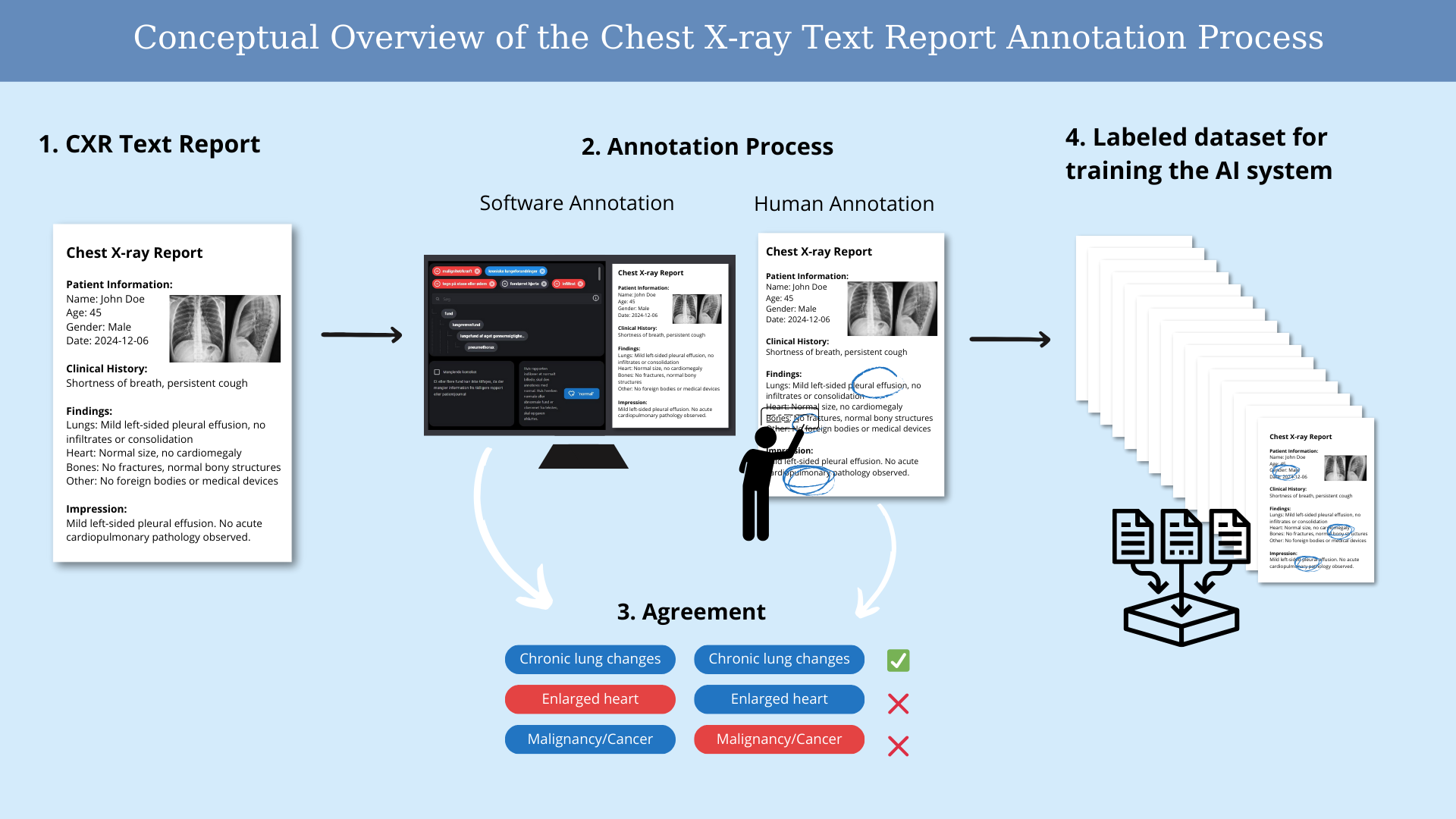
Performance in Annotating Chest X-ray Reports
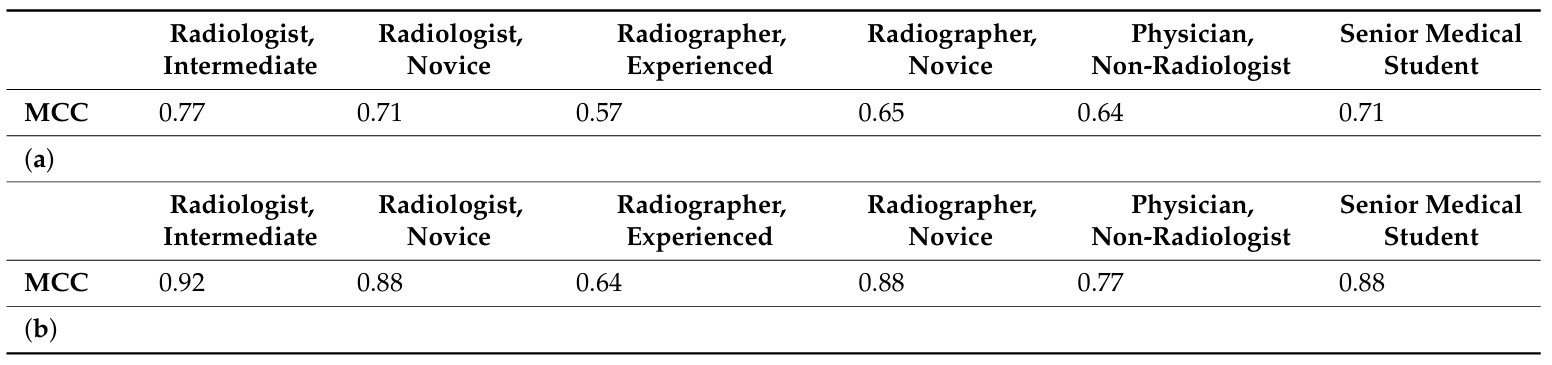