Feasibility of Multiparametric PET/MRI as a One-Stop Shop for RT Planning for Patients with HNC
Project by Anders Olin
This project evaluates the feasibility of using combined positron emission tomography and magnetic resonance imaging (PET/MRI) for radiation therapy (RT) planning in head and neck cancer (HNC). By generating synthetic computed tomography (sCT) images from MRI data using deep learning, both tumor delineation and dose calculations can be achieved. This method improves precision, particularly in soft tissues like those in the head and neck, while also reducing radiation exposure.
Project BackgroundHead and neck cancer (HNC) includes various cancers in the head and neck regions, where precise tumor delineation is crucial due to the proximity of sensitive organs. Current RT planning for HNC relies on positron emission tomography (PET) for identification of lymph node involvement and computed tomography (CT) for calculating radiation doses. However, CT has limitations in accurately delineating soft tissues, which can lead to uncertainties in target definition and impact treatment outcomes. Replacing CT with MRI could therefore establish PET/MRI as the optimal imaging technique for RT planning.
While MRI provides better soft tissue contrast, it lacks information about electron density, which is essential for accurate dose calculations. To address this limitation, a deep learning-based method has been developed to generate sCT images. The project aims to establish a PET/MRI scan protocol that enables PET/MRI as a comprehensive solution for RT planning by incorporating essential multiparametric MRI data and facilitating dose calculations through sCT.
Project PotentialThe deep learning method developed for sCT generation has demonstrated clinical suitability for both PET attenuation correction and RT dose calculations, representing a significant advancement toward integrating multiparametric PET/MRI as a one-stop shop for RT planning. Notably, the model has undergone external validation using a dataset from Guy's and St. Thomas' Hospital in London, further confirming its robustness and generalizability across different sites. While the method does have limitations, such as the need for larger datasets and adjustments for specific cases, the sCT images have proven clinical suitable, achieving dose calculations with only 1% difference from reference CT. This close alignment highlights sCT’s potential for enhancing RT planning.
Model Performance with Metallic Dental Implants
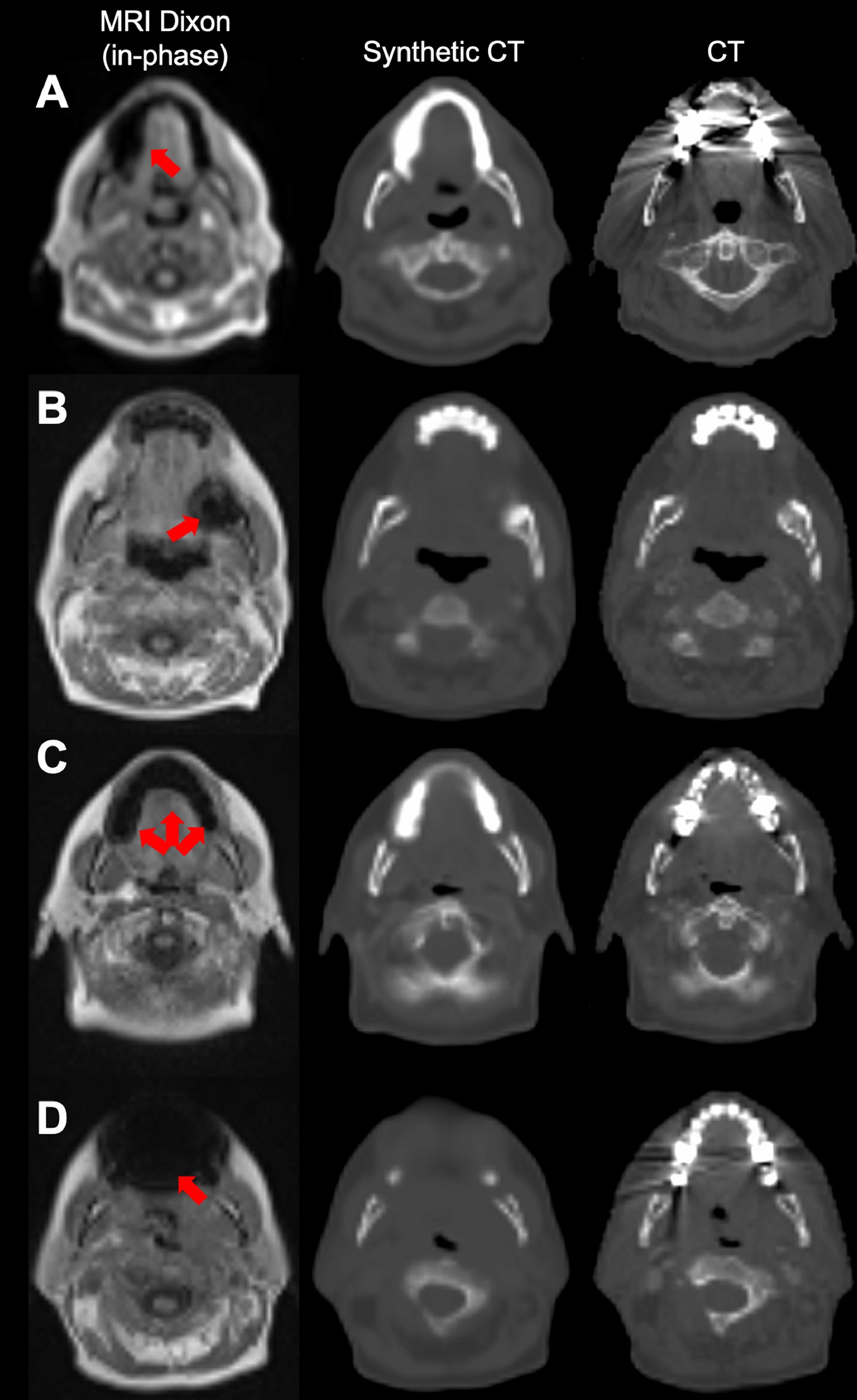
Figure 2. Cases illustrating the model's ability to handle metallic dental implants.
(A) A case from the external cohort, where the dental implant caused severe streaking artifacts in the computed tomography (CT) and a signal void in the magnetic resonance imaging without translating significantly into the synthetic CT.
(B-D) Cases from the local cohort, where dental implants only slightly affected the CT, but caused larger signal voids in the magnetic resonance images (MRI). For these cases the artifacts translated in varying degree into the synthetic CT images. Metal artifacts are marked on the MRI by red arrows.
Comparison of the Two Dose Calculation Methods (CT and sCT)
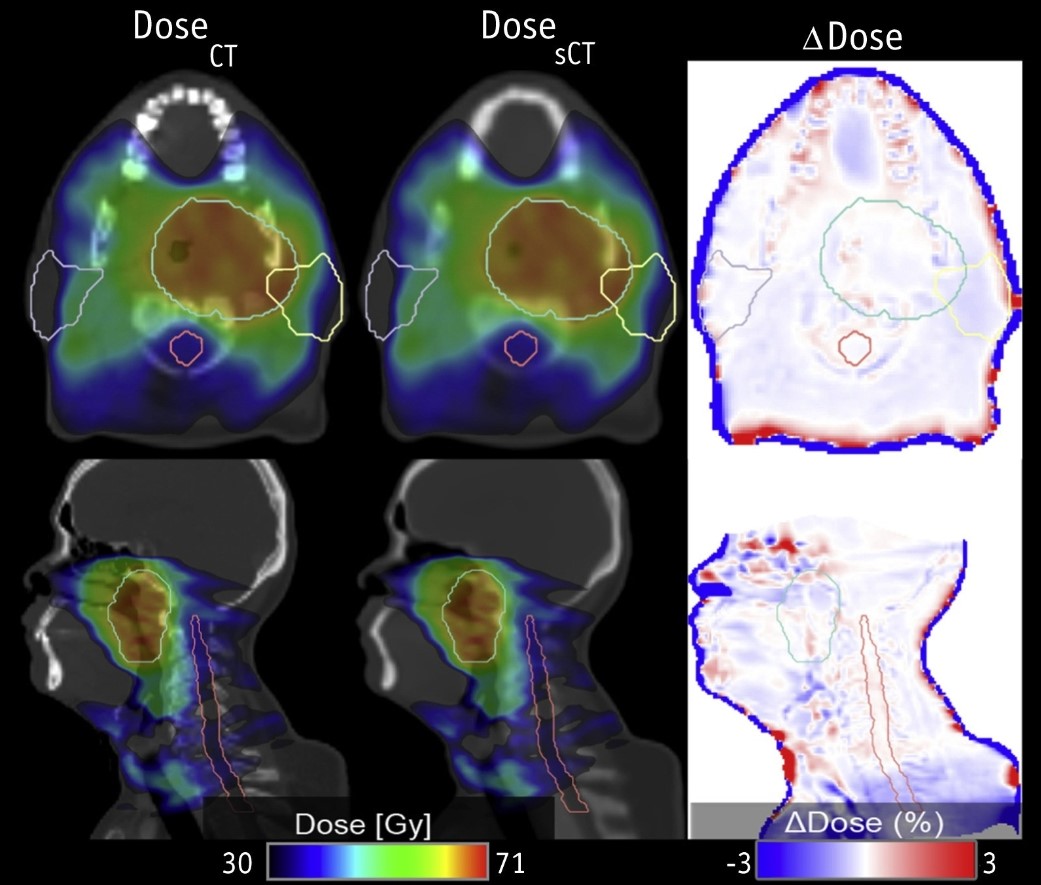