Automatic Brain Tumor Segmentation
Project by Peter Jagd Sørensen
This project explores two key advancements in brain tumor segmentation. First, it repurposes the BraTS dataset, originally designed for preoperative analysis, by introducing a two-label annotation protocol tailored for postoperative scans. This adaptation enables deep learning (DL) algorithms to segment tumors more accurately on postoperative MRI scans by excluding resection cavities from tumor regions. Second, the project evaluates the performance of the state-of-the-art HD-GLIO algorithm, focusing on its ability to segment contrast-enhancing (CE) and non-enhancing (NE) lesions in an independent set of postoperative MRI scans.
Project BackgroundAutomating brain tumor segmentation using DL algorithms has gained significant interest in recent years. The BraTS Challenge has been instrumental in this progress but is limited to preoperative MRI data, leaving a gap for postoperative scans. Most brain tumor patients undergo surgery, creating a need for annotated postoperative datasets, which are currently limited.
From another perspective, the project aims to assess the performance of the HD-GLIO algorithm on an independent dataset of postoperative MRI scans. The study evaluates how well the algorithm segments two types of lesions: contrast-enhancing (CE) lesions and non-enhancing (NE) lesions, providing insight into its applicability for routine clinical workflows.
By adapting the BraTS dataset with a two-label protocol, instead of a three-label protocol, and evaluating HD-GLIO, the project meets the gap between pre- and postoperative tumor segmentation, enabling DL algorithms to address real-world clinical needs.
Project PotentialThe project has the potential to advance postoperative brain tumor segmentation by addressing the lack of annotated datasets with a novel two-label annotation protocol. This innovation enhances deep learning accuracy, improves disease monitoring, and supports personalized treatment.
Although HD-GLIO shows strong potential for clinical use, particularly in segmenting larger tumors and NE lesions, it sometimes incorrectly identifies regions (see Figure 3). Addressing these challenges is key to refining models and ensuring clinical integration. This approach ultimately aims to streamline radiological workflows and improve patient outcomes.
Contact Information
Publications
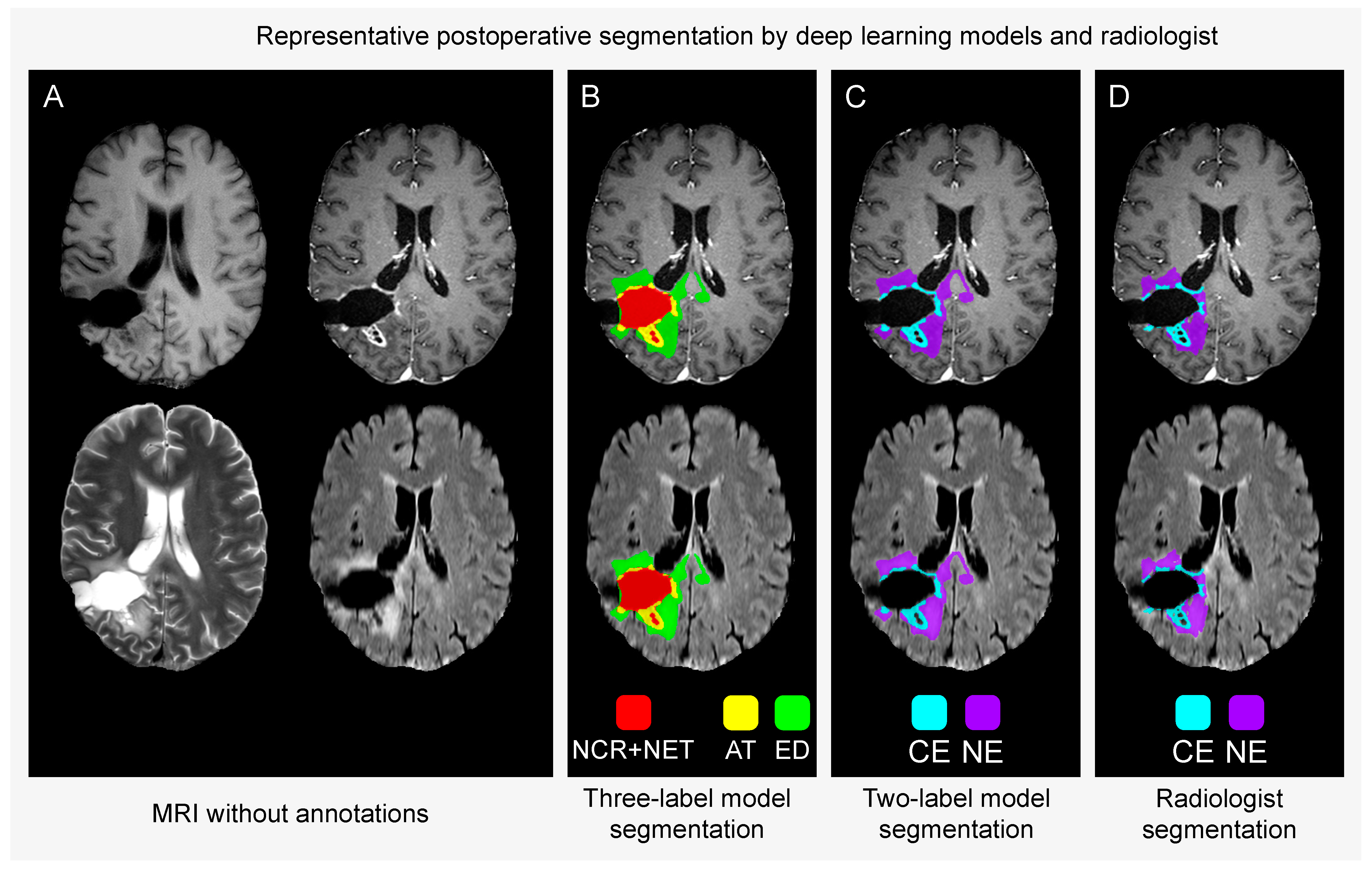
AT = active contrast-enhancing tumour
ED = oedema and infiltrated tissue
CE = contrast-enhancing tumour
NE = non-enhancing hyperintense T2/FLAIR signal abnormalities.
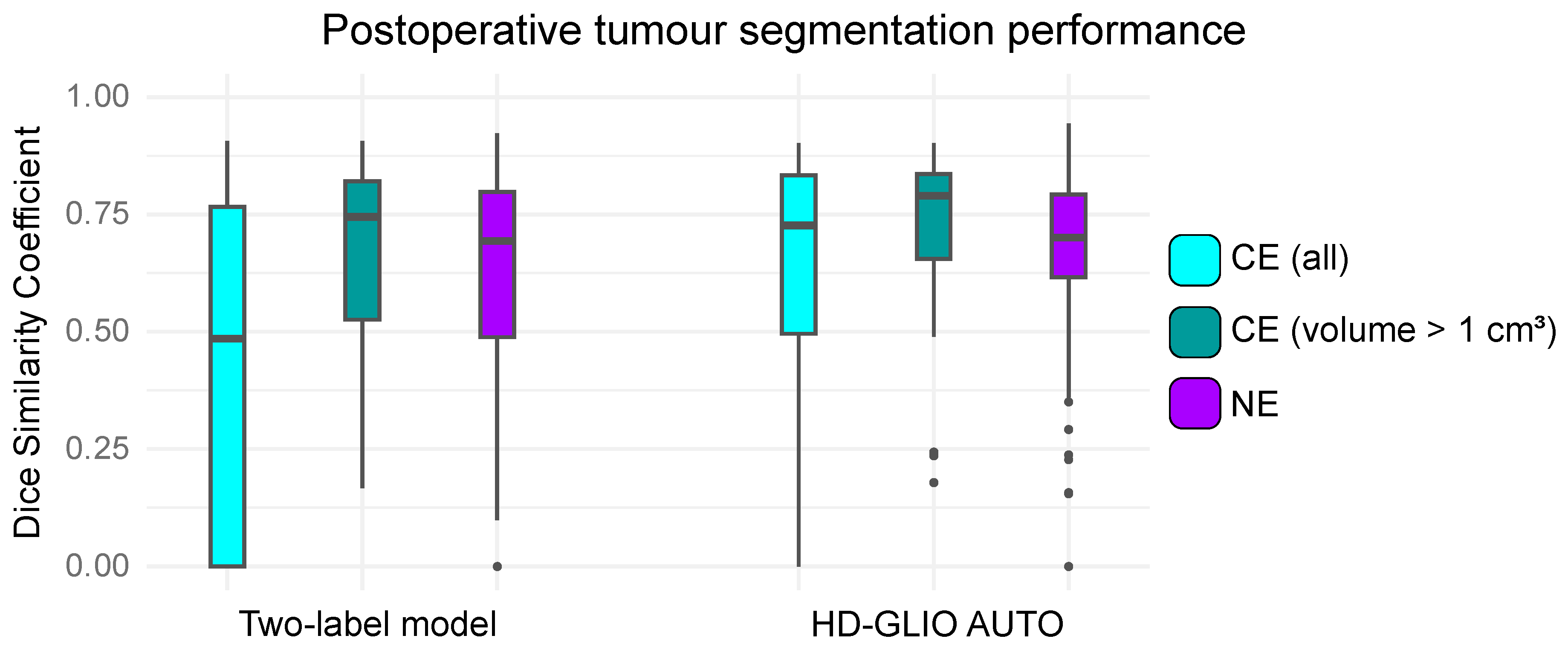
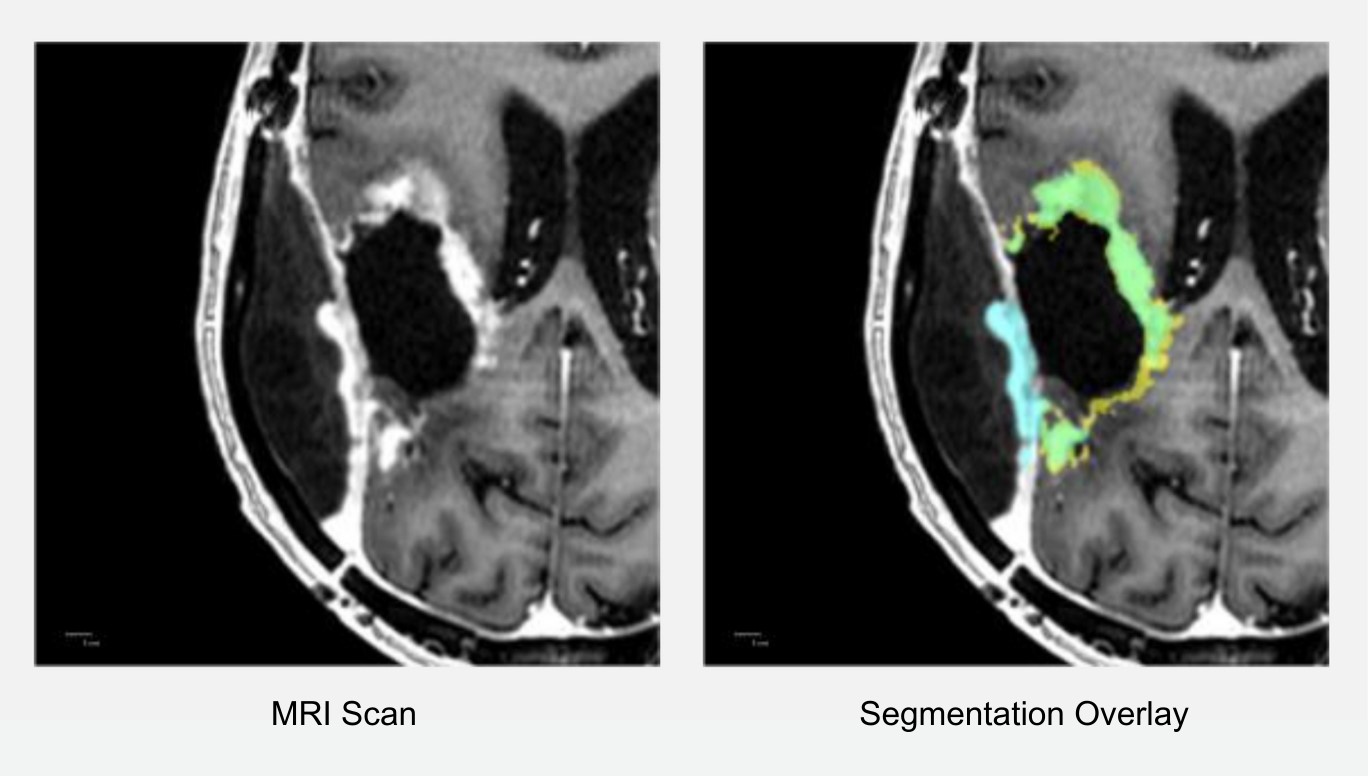